tfTarget Documentation
1 Login
The user needs to log in by clicking 'Log in' link at the top-right corner of the page. Having an account provides a number of benefits, and is free and easy.
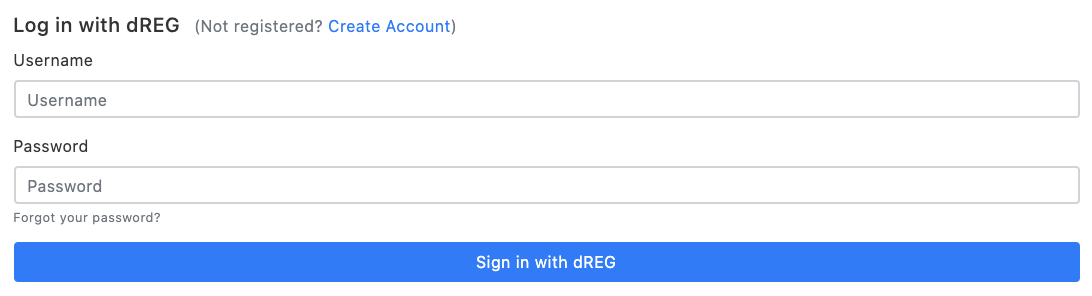
2 Create a new experiment
Select the tfTarget application on the Dashboard panel to create a data analysis for your data, as the following screenshot (Figure 2).
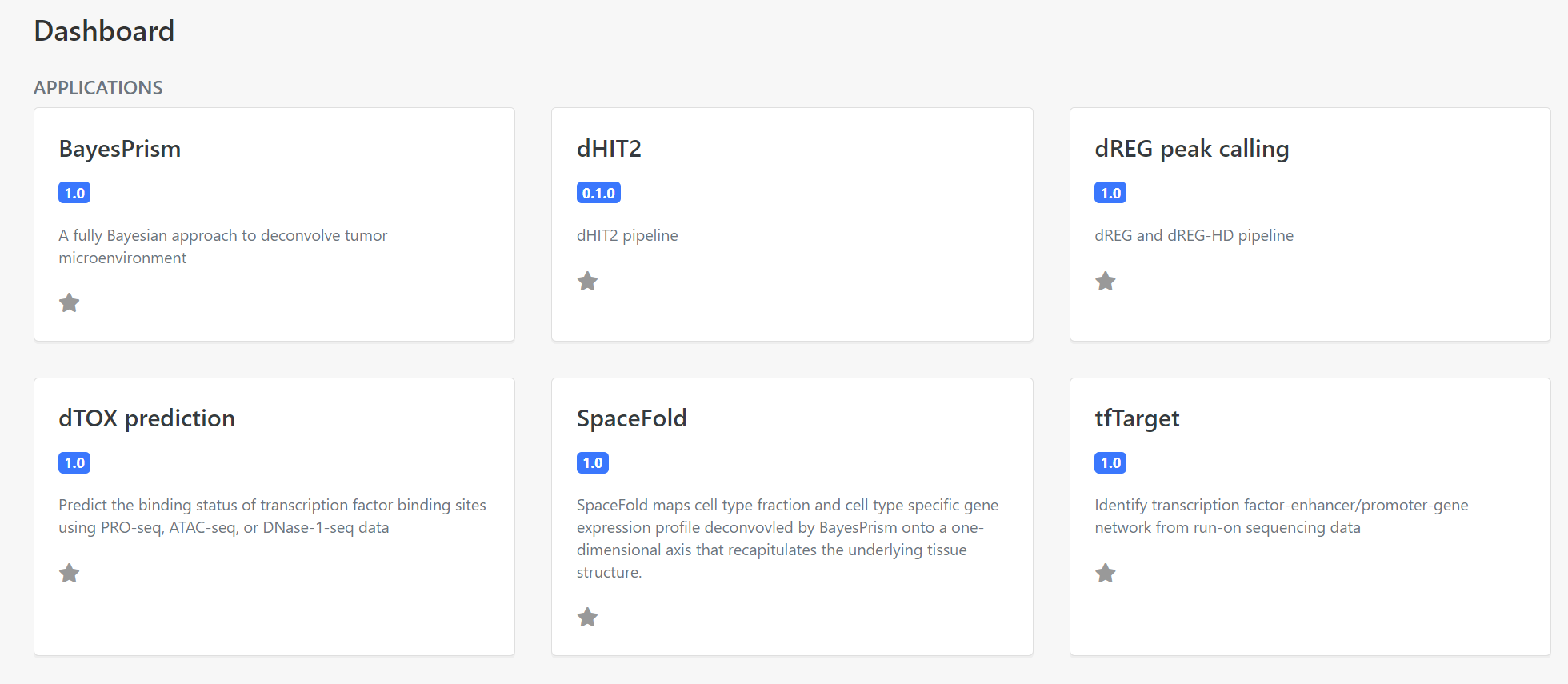
3 Set experiment name
Set "Experiment Name", and click "Add a description" to comment on the experimental setup page (optional). Choose the project that the experiment belongs to. By default, the "Default Project" is created and used.
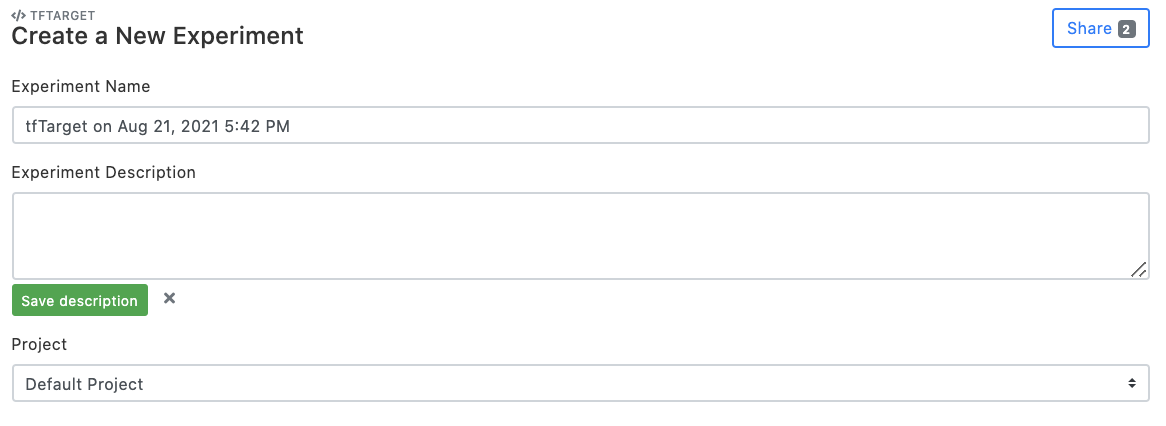
4 Upload bigWig files
There are two ways users may use to upload bigWigs.
(1) Click "Select files from storage" to choose existing files submitted for previous tasks, or
(2) click "Drop files here or browse" to upload new files from user's storage. Note that the bigWig files of run-on sequencing are strand-specific, and hence the ordering of bigWig files needs to be matched for plus and minus strands within each condition. Additionally, as tfTarget uses DESeq2 to model differential transcription and perform hypothesis testing, at least two replicates are required for each condition.
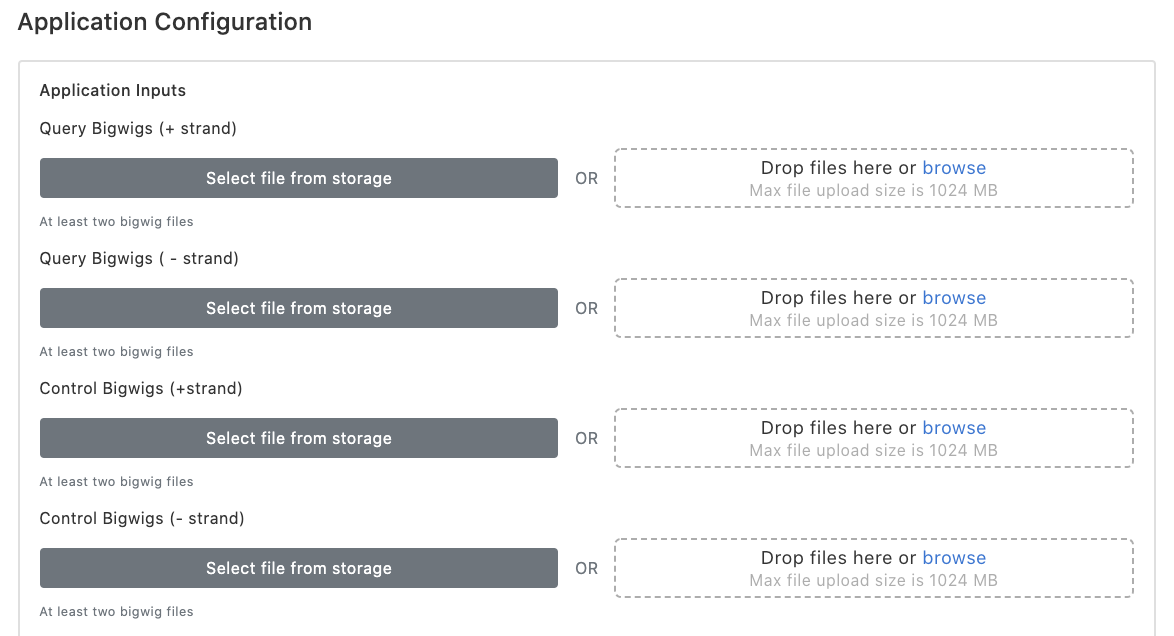
5 Set computing parameters
(1) Specify the genome assembly of bigWig files. Information of genome assemblies is required for defining gene bodies for quantifying their transcriptional activity and computing motif enrichment scores. Currently, only hg19, hg38 and mm10 are supported. If additional genome assemblies are needed, please submit a request to the admin.
(2) Specify the parameters for defining differentially transcribed TREs, genes, motif enrichment analysis, and the TF-TRE-gene network (Optional). Advanced users may refer to the flowchart of tfTarget and the input tab of this web page for the details of customizing parameters.
(3) Specify the prefix of the output files. This can help distinguish results from multiple experiments.
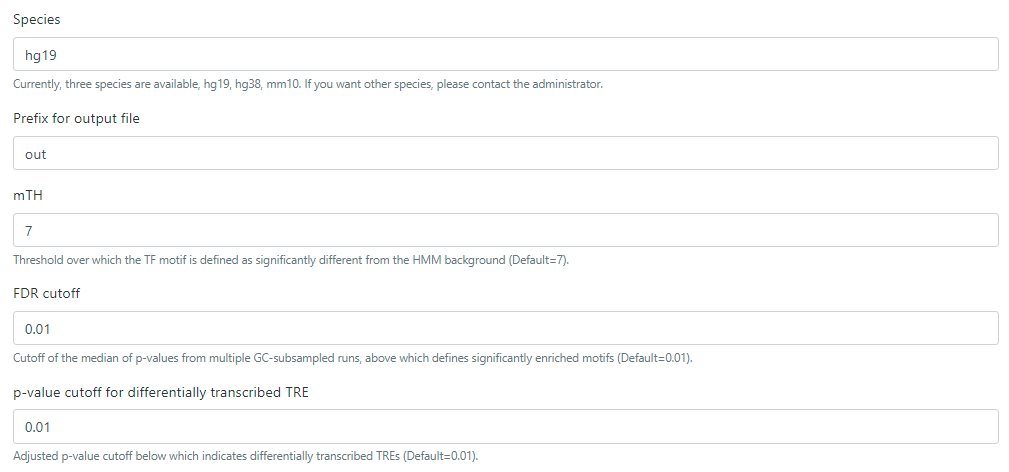
6 Submit the job
Once steps 1-5 are finished, proceed to "Save and launch". Input data and parameters will be submitted to the computing node of the XSEDE cluster via the dREG gateway server. Click the checkbox next to "Receive email notification of experiment status" if needed. dREG peak calling will be automatically performed on bigWigs merged for each condition. Upon launching, users will be directed to the "Experiments" page, shown in Fig. 4. A typical experiment usually finishes within 4 hrs. Users may view the progress by logging in and clicking the "Experiment" button on the left control panel at the dashboard.
7 Check the status
Users may view the progress by logging in and clicking the "Experiments" button on the left control panel at the dashboard. All experiments submitted are listed on this page.
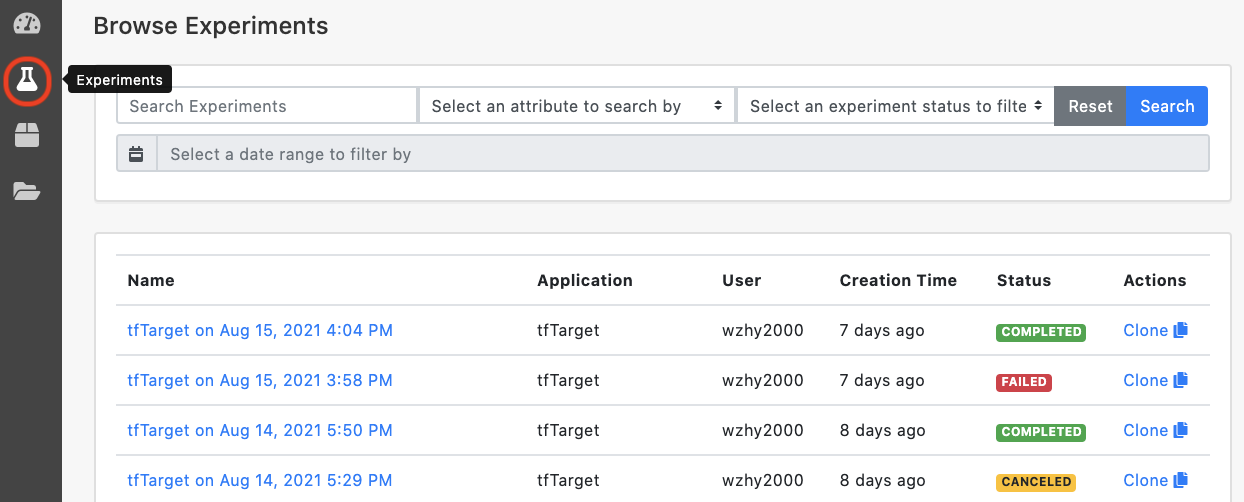
8 Check the results
Once a job is completed, the user can click selected tfTarget experiment and the website will jump to Experiment Summary page. All parameters used to set up the experiment are listed on this page. The user can also access output files of tfTarget stored in the ARCHIVE. Just click the ARCHIVE to check any single result file. A compressed file, including input bigWigs file set, two task log files and all result files, is also provided for users. Click Download Zip button to download a compressed file. The downloaded file with the 'tar.gz' extension can be decompressed by the 'tar' command, the file with the 'gz' extension can be decompressed by the 'gunzip' command in Linux.
In Safari, it could be problematic because Safari tries to unzip the compressed results automatically using a non-compatible compress method. Please check this link to disable this feature.
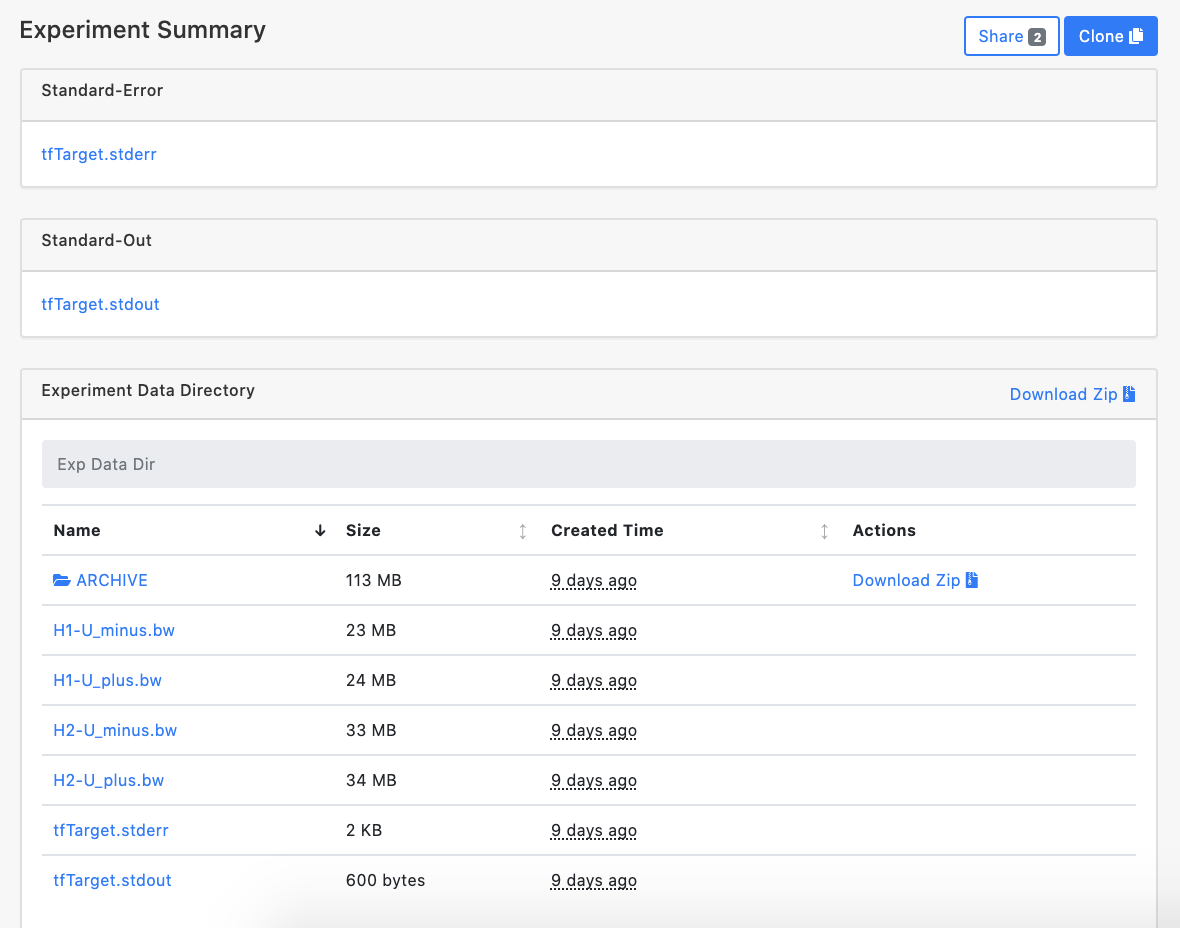
The input to tfTarget consists of two condictions' bigWig files which represent the position of RNA polymerase on the positive and negative strands. The sequence alignment and processing steps to make the input bigWig files can refer to the dREG service. tfTarget employs DEseq2 to detect the differential expression genes, so it requires at least 2 bigWigs for each condiction, treatment vs non-treatment, or query vs control, namely, each condition has at least 4 input files( 2 plus-strand bigWig files and 2 minus-trand bigWig files).
1 tfTarget parameter list
Note: The users can use the default parameters. This list provides the deatails which could be useful for advance users.
Parameter name | Description |
---|---|
P value cutoff for differentially transcribed TREs | Adjusted p value cutoff below which indicates differentially transcribed TREs. Used to define differentially transcribed TREs between two conditions. Default=0.01. |
P value cutoff for unchanged TREs | Adjusted p value cutoff above which indicates TREs that are not significantly changed between query and control. Used to define the background TREs for motif enrichment analysis. Default=0.1 |
GC subsampling cycles | The number of GC-subsampling cycles of motif enrichment tests to run. Higher numbers yield more robust estimates. Default=2. |
mTH | Threshold over which the TF motif is defined as significantly different from the HMM background. A Higher value yields a more stringent set with fewer number of enriched motifs. A value between 6 to 8 is usually a reasonable choice. Default=7. |
FDR cutoff | The cutoff of the median of p values from multiple GC-subsampled runs, above which defines significantly enriched motifs. Default=0.01. |
Distance cutoff | The distance cutoff (in units of base pairs) for associating TRE to the transcriptional start site of annotated genes. Default=50000. |
Closest N genes | Input the value to report only the first nth closest genes to the TRE. Default is 2. Set it to 0 to report all genes within the distance cutoff defined by the “dist” parameter. |
P-value cutoff for significantly differentially transcribed genes | Input the cutoff value to report genes that are only significantly differentially transcribed 1) with an adjusted p value lower than the cutoff specified, and 2) in the same direction as the regulator TRE. Default is 0.05. Set to 1 to report all genes regardless of significance in differential transcription. |
1 tfTarget output list
Note: All files below are stored in the "ARCHIVE" directory.
File name | Description |
---|---|
$PREFIX.query.minus.bw | Merged BigWig files (minus strand) of the query condition group. |
$PREFIX.query.plus.bw | Merged BigWig files (plus strand) of the query condition group. |
$PREFIX.control.minus.bw | Merged BigWig files (minus strand) of the control condition group. |
$PREFIX.control.plus.bw | Merged BigWig files (plus strand) of the control condition group. |
$PREFIX.control.dREG.peak.score.bed.gz | dREG peak calls of the control group. |
$PREFIX.query.dREG.peak.score.bed.gz | dREG peak calls of the query group. |
$PREFIX.merged.dREG.bed | Unified dREG peak regions merged across peaks identified in two conditions. |
$PREFIX_pval.upBd_0.01_pval.lowBd_0.1_mTH_7_rep_2_fdr_ 0.01_down.cor.heatmap.pdf | The heatmap of motif clustering for TF motifs enriched in TREs down-regulated in the query condition |
$PREFIX_pval.upBd_0.01_pval.lowBd_0.1_mTH_7_rep_2_fdr_ 0.01_down.motif.pdf | The dot plot of TF motifs enriched in TREs down-regulated in the query condition. |
$PREFIX_pval.upBd_0.01_pval.lowBd_0.1_mTH_7_rep_2_fdr_ 0.01_up.cor.heatmap.pdf | The heatmap of motif clustering for TF motifs enriched in TREs up-regulated in the query condition |
$PREFIX_pval.upBd_0.01_pval.lowBd_0.1_mTH_7_rep_2_fdr_ 0.01_up.motif.pdf | The dot plot of TF motifs enriched in TREs up-regulated in the query condition. |
$PREFIX.deseq.gene.deseq.txt | DESeq2 statistics of each annotated gene. |
$PREFIX.deseq.TRE.deseq.txt | DESeq2 statistics of each dREG region. |
$PREFIX.mapTF.pdf_dist_50000_closest.N_2_gene.pval_0.05.T F.TRE.gene.txt | A table listing the putative target genes for each TF enriched in differentially transcribed dREG regions, and their associated TRE. |
2 Interpret the results.
(1) Access the list of differentially transcribed TREs from the $PREFIX.deseq.TRE.deseq.txt text file. Each line is a TRE generated by merging dREG regions across two conditions.(2) Access the list of differentially transcribed genes from the $PREFIX.deseq.gene.deseq.txt text file. Each line is an annotated gene defined by the user's genomic assembly, while the statistics correspond to the DESeq2 outputs for transcription levels summarized over gene bodies. We use gene bodies as the surrogate for measuring transcription level. This avoids the complication of reads from the pausing site surrounding the promoters, and hence more accurately quantifies the amount of transcript being synthesized. Rows with all NA values are genes excluded from DESeq2 runs due to short gene length (<= 1kb).
(3) Access the TF motifs enriched in each condition from the motif.pdf files (Below).
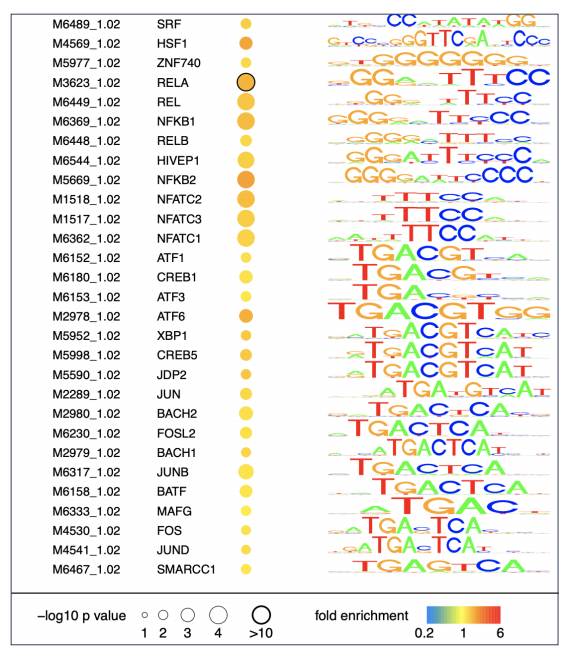
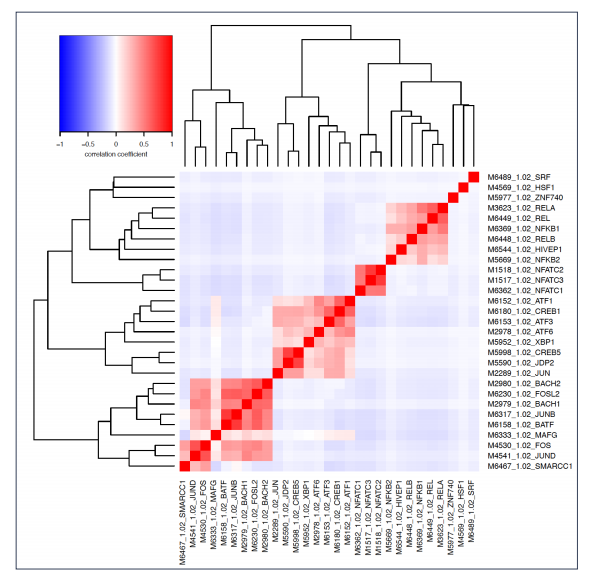
dREG Gateway is online service that supports Web-based science through the execution of online computational experiments and the management of data. The items below are trying to answer qustions from the users
Q: How should I prepare bigWig files for use with the dREG gateway?
A: Information about how to prepare files can be found here .
Q: How should I do when I meet the computational failure in the dREG gateway?
A: There are two types of error you may have, we explain how to identify your error and how to handle it here.
Q: Which browser works well with the dREG gateway?
A: We have tested in the Firefox, Google Chrome and Safari so far. For IE (version 10 or 11) and some version of Safari, you maybe have trouble showing sequence data in WashU genome browser. For Safari users, please read next Q&A.
Q: What should the Safari users be aware of?
A: By default, Safari unzips a zip file automatically when you download it. However dREG results are compressed by the 'bgzip' command which is not compatiable with the Safari method. It would be probelmatic when you download dREG results. Please refer to this link to disable this feature in Safari and then download the compressed results from dREG gateway. Secondly, when you click the genome browser link, please use the Left-Click, don't use Right-Click menu and the menu option "open a new tab".
Q: What types of enhancers and promoters can be identified using the dREG gateway?
A: As a general rule of thumb, high-quality datasets provide very similar groups of enhancers and promoters as ChIP-seq for H3K27ac. This suggests that dREG identifies the location of all of the so-called 'active' class of enhancers and promoters.
Q: Will the dREG gateway work with my data type?
A: The dREG gateway will work well with data collected by any run-on and sequencing method, including GRO-seq, PRO-seq, or ChRO-seq. Other methods that map the location of RNA polymerase genome wide using alternative tools (for example, NET-seq) will most likely work well, but are not officially supported.
Q: Will the pre-trained models work using data from my species?
A: Models are currently available only in mammalian organisms. The length and density of genes, which vary considerably between highly divergent species, affects the way that a transcribed promoter or enhancer looks. For this reason, models can only be used in species. We are working to create models in widely-used model organisms, including drosophila and C. elegans.
Q: How deeply do I need to sequence PRO-seq libraries?
A: Sensitivity is reasonable at ~40 million mapped reads and saturates at ~100 million mapped reads. See our analysis here: supplementary figure 3 in dREG paper.
Q: How long do my data and results keep in the dREG gateway?
A: One month.
Q: How to I cite the dREG gateway?
A: Please cite one of our papers if you use dREG results in your publication:
A: Please cite one of our papers if you use dREG results in your publication:
(1) Wang, Z., Chu, T., Choate, L. A., & Danko, C. G. (2019). Identification of regulatory elements from nascent transcription using dREG. Genome research, 29(2), 293-303.
Q: Do I have to create account before using this service?
A: Yes, this system is supported by an NSF funded supercomputing resource known as XSEDE, who regularly needs to report bulk usage statistics to NSF. Nevertheless, data that you provide are completely safe.
Q: How do I know the status of the computational nodes?
A: Since we can't update this web site very often, the gateway status is updated here on the dREG page based on the notifications of the XSEDE community.
Q: Who do I thank for the computing power?
A: This web-based tool is powered by SciGaP and Apache Airavata and the GPU servers are supported by the XSEDE.
Q: I have another question that is not on this FAQ. How can I contact you?
A: Yes, please contact us with any questions! Zhong(zw355 at cornell.edu). Charles(cgd24 at cornell.edu).